-
Charlotte Hausman authoredCharlotte Hausman authored
How Workflows Run in the DSOC or NAASC
Introduction
Our workflows are executed by HTCondor, which is a kind of job scheduler. Unlike other job schedulers, HTCondor is designed to support a heterogeneous cluster and puts more emphasis on the reliability of the processing than the speed.
HTCondor does lots of magic behind the scenes, but the interface is simple: you make a directory with a job description file in it and submit it. The machine you started at is the submit host. HTCondor queues the job and eventually matches it up with an execution host. The directory is copied to the execution host and according to the job description, an executable is run. When the job completes, HTCondor copies the results back to the submit host. During the interim, a log file has been updated with everything that has occurred. Basically, you can pretend that it works as in this diagram:

Labels
HTCondor allows you to specify additional requirements for a job. These requirements are kind of like labels that the execute host should possess. We currently use three:
-
HasLustre
: the execute host must have access to the Lustre network file system -
CVPOST
: the execute host must be in Charlottesville -
VLASS
: the execute host must be a VLASS-sponsored node
These labels do not form partitions; for instance, some VLASS nodes have Lustre and some do not.
Data Locality
To understand the data locality situation here, there is a table maintained by the SCG and CIS and the following diagram illustrates the situation:
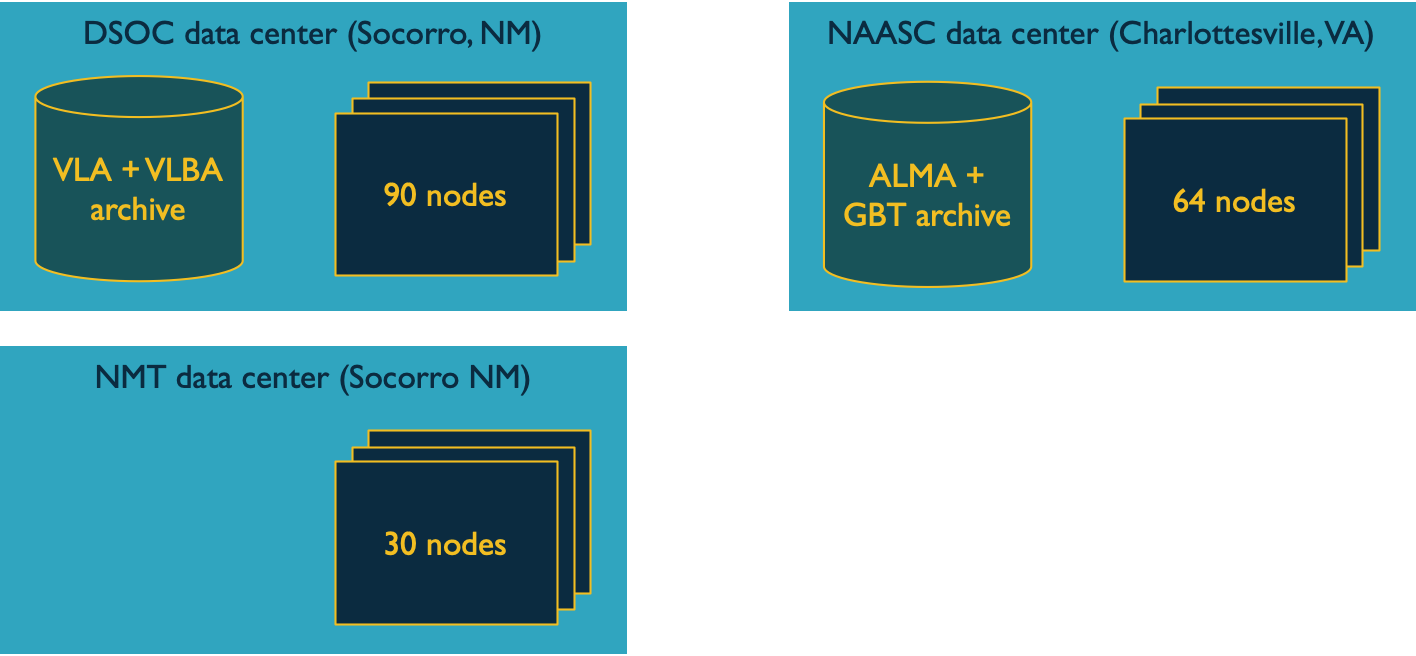
We have data in NGAS both at the DSOC and at the NAASC. The data at DSOC are VLA and VLBA; at the NAASC they are ALMA and GBT. There are other datacenters besides these two, like NMT, or hypothetical datacenters like UW Madison, AWS or Xcede. These datacenters may or may not have something like locality to various data—e.g. NMT nodes are not physically far from the DSOC nodes (I don't know whether the link is faster to NMT or not) but the UW Madison and AWS clusters are not effectively local to anything. It's important for jobs to run local to the data they need—it's cheaper to move the computation to the data than the data to the computation.
An important attribute of HTCondor is that the submit host and the execution host do not have to belong to the same datacenter or on the same network. The Workspaces system relies on this fact by assuming that there can be a single workflow service running on a single submit host, and this is enough to arrange for jobs to be executed with locality to ALMA or VLA data—more obviously, to submit jobs to be executed within the DSOC or NAASC datacenter, or even others we don't yet possess.
Data Staging
HTCondor
A traditional supercomputer would be comprised of identical nodes. Because HTCondor doesn't require that the nodes be similar to each other, it creates what's called a heterogeneous cluster. The main thing HTCondor does is simply making jobs come to life in directory with the files mentioned in the job description. A substantial subsystem in HTCondor concerns this data staging, meaning, moving files around for you, and there is a plugin architecture to make this process extensible. The result is that there are two approaches to staging data for your job:
- Put your data in the directory you submit from and inform HTCondor; HTCondor will copy it to the job for you somehow
- Your job can retrieve its own data when it starts
By default, there is no way of dictating which network devices are used. This prevents us from using HTCondor's data staging for bulk data. However, the Scientific Computing Group has written a plugin which we use to transfer data efficiently over the correct network interfaces, called condor-transfer-plugin.
NGAS
Most jobs use archived data as their starting point. We have a program called
:doc:`productfetcher <../tools/productfetcher>` whose job is to copy files out of NGAS.
NGAS has a direct-copy plugin we authored to speed retrieval from NGAS to Lustre. Using
the direct copy plugin utilizes Infiniband and is thus 10× faster than streaming the data
out over the 1 Gbit ethernet. This introduces a dependency on the job being able to see
the same Lustre as the NGAS cluster, but this is handled by the aforementioned
HasLustre
label in the job description file.
Follow-on requests
A significant functionality of the capability system is that it permits "follow-on requests." A follow-on request uses the result of another request. The exact stage of the earlier request's lifecycle doesn't matter, as long as it has not been cleaned from disk. This introduces a second way for jobs to get their initial data besides the datafetcher/NGAS. The request results are kept around in some accessible location for later requests. Workflows that start from earlier requests shouldn't need to be structurally different from workflows that start with archived data.
This functionality is an important aspect of the workspaces system but it remains currently unimplemented.
Workflows Plan
Cluster setup
The path forward here seems quite simple:
- SCG sets up a node locally which pretends to be a NAASC/ALMA-local node
- SSA conducts tests using that node
- Meanwhile, SCG locates a node in the NAASC for further testing and sets it up
- SCG migrates hosts shipman and hamilton to become submit hosts for the production HTCondor cluster
Science Data Flow
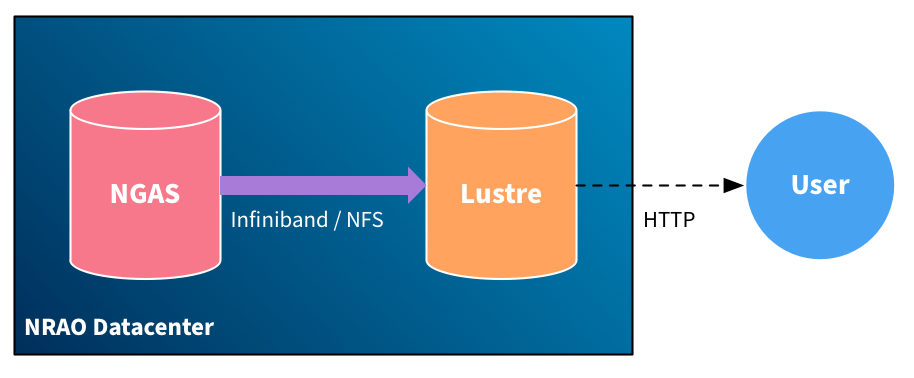
In the simple download case, data is retrieved from NGAS to Lustre, from which it is served to the user over HTTP.
For reprocessing, the most general case is one in which the data is retrieved to a foreign datacenter for processing and then copied back.
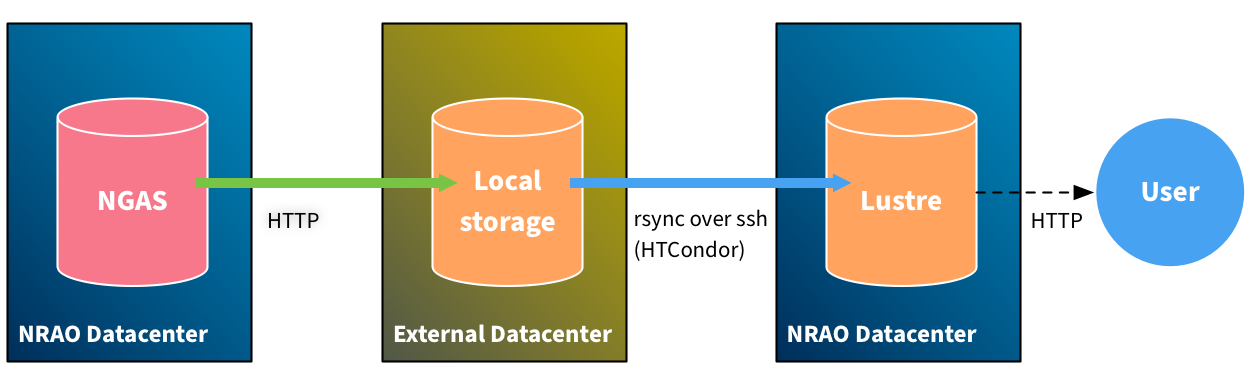
There is a lingering question here about how to fetch data from NGAS in a remote location. The streaming system that NGAS offers is going to use the HTTP protocol and is unable to benefit from Infiniband. A better alternative would be implementing Globus/GridFTP as an NGAS plugin. An intermediate solution might be having a local job do the staging of data from NGAS to Lustre prior to executing the cluster job.
To make reprocessing jobs relocatable, they must always behave as if they are running in a local datacenter, but when HTCondor jobs are scheduled inside the NRAO datacenter, their local storage may actually be on Lustre (depending on the node), and the data flow will reduce to something much more similar to the first diagram:
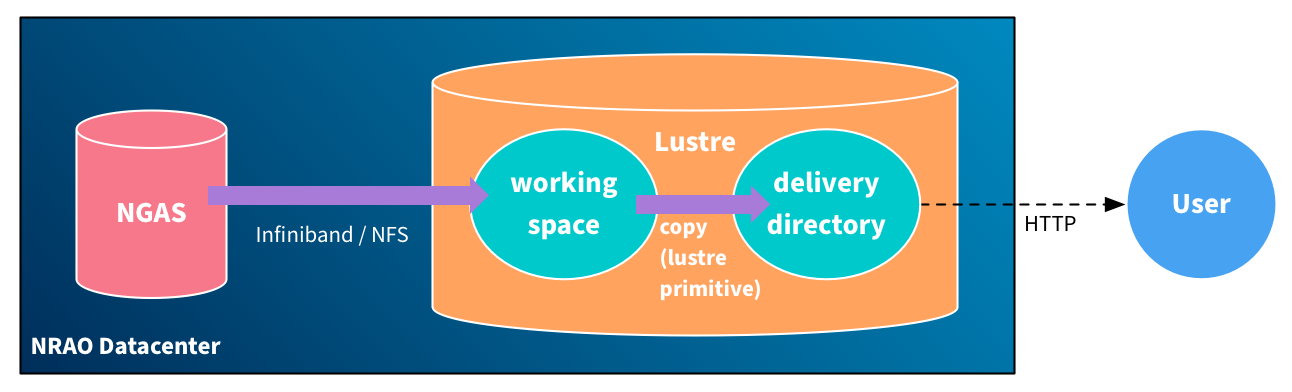
This is because the data fetcher will preferentially use the local copy option when it is available, and rsync can be made to use a local cp if it is possible instead of the streaming network mechanism.